May 19, 2022
Podcast
Modal Technology Corporation and McGill University Health Centre Research Institute (MUHC-RI) were recently interviewed by Maria Palombini, Director of IEEE SA Healthcare and Life Sciences. Maria is host of the Re-Think Health podcast, which is an interview-style podcast where global healthcare stakeholders, i.e., technologists, researchers, clinicians, patient advocates, regulators, and more, re-think the approach to healthcare, from therapeutic discovery through bedside practice, utilizing new technologies and applications.
In the podcast, Nathan Hayes, CEO and Founder of Modal Technology Corporation, and Dr. Anthoula Lazaris, Scientific Director at MUHC-RI, discuss their research collaboration in precision oncology, which has led to the development of a novel method for biomarker identification and ranking from non-invasive blood liquid biopsy samples in the early diagnosis of metastatic liver cancer. The discussion focuses on how the use of ALiX™, the world’s first non-statistical artificial intelligence (AI) tool, successfully discovered a predictive biomarker profile, and how the unique capability of ALiX to rank biomarkers from least to most relevant, a feature not available anywhere else, is overcoming scientific obstacles on the critical path to the commercialization, in the form of a point of care lab kit.
Listen Now
Re-Think Health Podcast, Season 3, Episode 2: Riding the Third Wave of AI for Precision Oncology
What is the “third wave of AI” and how is it different than the first and second waves? Anthoula Lazaris, Scientific Director at McGill University Health Centre Research Institute and Nathan Hayes, CEO and Founder at Modal Technology Corporation join the podcast to discuss the application of the “third wave of AI” with real-world data and practice to realize the potential for precision oncology and improve patient outcomes.
About the Speakers
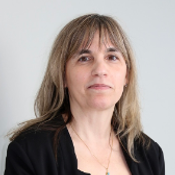
Anthoula Lazaris
Anthoula Lazaris, Scientific Director at McGill University Health Centre Research Institute, is a scientist with over 26 years combined experience in academia (McGill University) and biotechnology/industry, in management and senior-level positions with a demonstrated history of working in the hospital and healthcare industry.
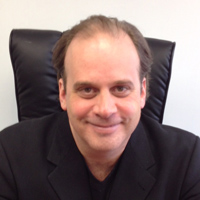
Nathan Hayes
Nathan Hayes, CEO and Founder at Modal Technology Corporation, is an entrepreneur, mathematician, and software architect with more than 20 years of experience working in these combined fields. Hayes specializes in the applied science of modal interval analysis to the fields of artificial intelligence, machine learning, and high-performance computing.
About the Host
As the leader of IEEE SA Healthcare and Life Sciences, Maria Palombini works with a global community of multi-disciplinary stakeholder volunteers who are committed to establishing trust and validation in tools and technologies that will change the approach from supply-driven to patient-driven quality of care for all. Her work advocates for a patient-centered healthcare system focused on targeted research, accurate diagnosis, and efficacious delivery of care to realize the promise of precision medicine.
Transcript
Click here for a full transcript of the interview.
Maria Palombini
Hello everyone! I am Maria Palombini, and I am Director and Lead of the Healthcare and Life Sciences practice here at the IEEE SA. And I’m also your host for the Re-think Health Podcast, Season 3: AI for Good Medicine.
The Healthcare Life Science practice is a platform for bringing multidisciplinary stakeholders from around the globe to collaborate, explore, and develop solutions that will drive responsible adoption of new technologies and applications leading to more security protection, and sustainable equitable access to quality of care for all individuals. Yes, this is an ambitious goal, but a much necessary one. The Re-think Health Podcast series seeks to bring awareness of these new technologies and applications with a balanced understanding in how to use them, how to be applied, and where potentially they may be need for policy standards or whatever it takes to drive more trusted and validated adoption to enable better health for all.
We have previous seasons available on Podbean, iTunes, or your favorite podcast provider. AI for Good Medicine Season 3 will bring a suite of multidisciplinary experts, technologists, clinicians, regulators, researchers, all with the goal to provide insights as how do we envision artificial intelligence or machine learning or any other deep learning technology, delivering good medicine for all? Naturally, we all want good medicine, but at what price? Especially, in terms of trust and validation and its use. So as healthcare industry stakeholders, we are not looking for the next frontier of medicine if it’s not pragmatic, responsible, and can be equitably valuable. So in this season, we go directly to all these experts and we try to get to the bottom of it and make real and trusted impact improving outcomes for patients anywhere from drug development to healthcare delivery.
So the question is: will AI, ML, deep learning cut-through the health data swamp for better health outcomes? With that, I would like to welcome Anthoula Lazaris, Scientist at the Research Institute of McGill University Health Center, and Nate Hayes, Founder and CEO of Modal Technology Corp. In this discussion, they’re going to talk to us about the third wave of AI for better patient outcomes and potentially realizing precision oncology. This is a fascinating case study. From the minute I heard about it, I was very excited about it, and I think it really shows how we can start to move the needle forward.
We are now on segment 1. We like to humanize the experience for our audience and we want to humanize the people behind the microphones. So a little bit about Anthoula. She has more than 26 years combined experience in academia, McGill University, biotechnology industry and management in senior-level positions with a demonstrated history of working in the hospital in the healthcare industry. She has been at the Research Institute of McGill University Health Center for a little over 11 years, focusing on bringing precision oncology to patients through clinical research projects.
Some of her career highlights include being part of the team making Nexia’s IPO, the largest public offering in life sciences in Canada, up to 2002. She was the first to demonstrate that a translation initiation factor can act as a proto-oncogene. The work was published in Nature.
And, Nate. He’s an entrepreneur, mathematician, and software developer who has been instrumental to the development of Modal Interval Arithmetic and served six years as a committee member of the IEEE 1788 Standard for Interval Arithmetic.
In addition to his executive leadership at Modal, he is Co-founder and board member of RISC AI, Inc.
So, Anthoula, Nate. Welcome to Re-think Health.
Anthoula Lazaris
Thank you, Maria, for having us as well and giving us the ability to present our collaborative project.
Maria Palombini
Oh, this is very exciting. I’m so interested to get to the nuts and bolts of this interview.
Okay, so I’m going to start with you, Nate. Maybe you could share a little bit with us what is a ALIX, A-L-I-X and what is the fundamental difference between a third wave versus a second wave AI tool? I mean, we’re just getting onto AI and you’re talking third wave, so obviously you’re already light years in front of us.
Nathan Hayes
No, that’s a great question and maybe to provide a little context here, I’ll even rewind and go back to the beginning and give an overview of where things started in the first wave back in the 1970s to 1990s, kind of roughly is the time period of what we would call the first wave of AI. These early AI systems were very good at reasoning. You know, playing chess or checkers, for example, but they didn’t really have an ability to learn because the way that they were developed is typically humans would program these systems with a set of rules, like what are the rules for chess, for example. And then the computer could use those rules to reason about the chessboard and act as an artificial opponent, for example, in the game. Things evolved roughly in the timeframe of about the turn of the century here, 2000 to present, I think, most people would characterize or agree that we’re still primarily in the second wave of AI and the main distinction here between the first wave is that in the second wave, the machines have actually become good at learning. So they not only can reason, but they can actually learn how to do something.
Machine learning, for example, is looking at a pile of photographs and saying, is it a cat or is it a dog. The machine by analyzing a large test or a training dataset of images, it can actually learn how to interpret the images and then after the training is complete, you can put in new images that the computer wasn’t aware of or that it didn’t get the seed during the training time, and it will then predict. It’ll say, oh, I think this is a cat or I think this is a dog.
So fundamental to this concept is you’ve got a training process for the second wave machine learning or artificial intelligence and in that training process, you’re analyzing very large sets of data so that the machine can find patterns in the data and it can learn. And then after the training process is finished, then you have deployment out into the field and the machine will then, what we call inference, or make predictions based on the results of the training process.
And from a mathematical perspective, what’s really going on here is this learning process is a very complicated non-linear global optimization problem. So that is the main characteristic of how these machines learn under the hood in a mathematical perspective. And the other characteristic that I think really defines the second wave that we’re currently in is that when the current algorithms and the current computers and methods that are used to solve this optimization problem are primarily statistical in nature.
The reason this is important to understand is that since everything is statistical, and confidence can only be measured for example, in terms of probabilities, you’re never really completely sure exactly where you stand in terms of how well of a job you’ve done with the machine. And in that sense, a lot of people have talked about using these second wave tools that it’s like working with a black box.
So as we talk about entering the third wave, the primary difference here from the second wave AI is that in the third wave, the machines become excellent at learning. And in addition to that, the machines begin to provide explanations. We’re overcoming that black box capability and we’re providing a more clear and concise and intuitive answers to the humans that are trying to work with the AI in terms of understanding how the machine goes about making certain decisions or predictions.
So this is what is very broadly called explainable AI since it’s kind of a new concept, there’s really a lot of different definitions and a lot of different groups that are starting to work in the third wave may have different definitions of what explainable means, but explainable AI from our perspective means that because of this new approach that we are using with the ALIX training method, which is built on this Modal Interval Arithmetic and it’s a completely different algorithm or method, if you will, than anything else that’s currently out there. The thing that is different is it’s not a statistical approach to training or solving that optimization problem. And so in that regard, we’re getting rid of all of these probabilities. We’re providing guarantees and repeatable results and answers through this process and through this unique capability, we’re also opening up that black box and providing a guaranteed view or answer to how did the machine, for example, arrive at this particular conclusion in terms of making a prediction that a picture contains a cat versus a dog or if in terms of healthcare, what we’re talking about today, is a patient healthy or they have a particular diseases.
Maria Palombini
Great, Nate. Thank you. Anthoula. Obviously Nate set the foundation for us on the technology. You know, we talk to a lot of clinicians and researchers and sometimes they’re like, oh, I don’t know about this AI thing when we’re talking about research. Can you give us a little insight about the case study, what you were going for in your research, and then at the end of the day, why you chose to move forward with a cutting edge AI tool, such as ALIX for this precision oncology research.
Anthoula Lazaris
When we talk about precision oncology, we’re talking about not treating just the disease, but treating the patient who has the disease. So really identifying unique features within that patient’s cancer. As we identify these unique features in the cancer, new technologies have evolved. For example, liquid biopsies, we hear about liquid biopsies.
This is what we’re doing here is we no longer need a sample of the tissue from the patient, which is very invasive. Instead we’re using a liquid form in terms of it could be blood, urine, saliva are just three examples. So with respect to the project that we have with Nate and where we started it. So with a basis, looking at precision oncology, really trying to focus on individual patient care and applying liquid biology, which is really in our case, looking at components within the blood that are either shed by or changed by the cancer.
The work that we do in our lab is focusing on colorectal cancer liver metastasis. We looked at the tissue and we identified markers that could predict a patient’s response to treatment, but we literally need tissue for this, which is not always practical when it comes to getting biopsies from patients. So the starting point of this project is we already had some predefined specific features within the tissue that we now said, well, let’s go into a liquid biopsy and see if we can identify these features in the blood and in essence, identify which patients will respond to treatment and which patients will not respond to treatment. And for this in the blood specifically, you hear a lot about circulating tumor DNA, where they’re looking at genetics. We took a different approach. We’re looking at these vesicles that are secreted by multiple different cell types and we looked at the proteins within these vesicles. So the starting point, the large amount of data we collected was vast amount of proteins from mass spectrometry data on the blood of two different populations of patients, those that do respond to current treatment and those that did not respond.
When we first met Nate, which actually was brought to our team from our business development office. So, as you can see, there’s a lot of multidisciplinary going on here. He presented ALIX to our team and we were really surprised that this type of analysis program, you call it third wave, actually existed. And at the time, Nate just referred to the basic bioinformatics tools, which really rely on statistical significance.
That’s a key feature here, I believe, because when we talk about statistical significance, so we pulled out based on our tissue and even looking at the blood proteins, we use bioinformatic tools on all the proteins we’ve pulled out of the blood. And we found over 50 proteins that looked like they were different between the two patient populations. But we had no idea which ones were important, which ones were not important. We couldn’t rank them to identify. So we’re screening now looking at 50 different proteins, which is very time consuming. So we were intrigued that ALIX could actually develop a signature for us. And also rank the signature and the biomarker found in the blood according to importance in answering our question, what will lead to a patient not responding to treatment versus a patient responding to treatment.
Maria Palombini
That’s fascinating. So much going on in the world of oncology research and to start to get at that level is critical, but really just amazing.
Anthoula Lazaris
First of all, we were surprised from the start in terms of what Nate and his team had developed in terms of, I wouldn’t even call it a software, I’d call it ALIX. So ALIX is our friend. The main outcome is we generated a signature that was able to tell us which patients would respond to disease, which patients will not respond to disease. And importantly, like I said, it was able to rank them as relevant and irrelevant.
The other thing that came to our attention was the way ALIX worked. So I’m a molecular cell biologist. I am not a mathematician or an AI person. What I had to understand from the beginning is that ALIX is driven by a multiplex analysis. We’re not looking here at identifying individual biomarkers. So it had to be clear from the beginning when we were first discussing with Nate and his team that we’re not looking at an individual biomarker, we’re not looking for a target for new drug here. That was not the goal of the project and we had to keep our focus like that.
Once we saw the signature, we said, okay, let’s apply our biological knowledge and look at different pathways and see what pathways are up or down regulated. It wasn’t that simple. Applying the biology to ALIX’s signature was novel.
It’s one thing to find a solution, i.e. the signature. It’s another thing to actually understand the solution. So we only had half the battle won at this point. So what we eventually through repetitive meetings and discussions with Nate, and I think that’s, what’s really important in this type of collaboration is Nate comes at it with his mathematical background and AI background. We were able to communicate, we’re able to understand each other’s languages. Whereas I was coming more from a biological sciences background, but through discussions, we were able to realize that ALIX’s solution was really telling us a whole body’s response to the disease. So it’s not just the tumor itself, tumor cells in the blood that people often find, et cetera. We’re not looking at that. What ALIX has identified for us is the body’s physiological response to the cancer. This is new. We had to figure out ways of trying to understand. So how do we now look at the whole body as a whole to understand what the signature means?
In essence, we had two major findings. One is we’ve developed a signature, which we will now move on to try to bring it into clinical practice. But again, that’s longterm still. And second is understanding what the solution ALIX is providing and how we could use that to better understand the physiology of the human body.
Maria Palombini
Wow, it’s unbelievable. I think that’s just amazing. I guess that’s when they say when you’re putting data to good work. One of the benefits of having both the technologist and the researcher on these kinds of interviews is that you can get both perspectives at the same time. So first I’ll start with you, Nate.
ALIX is scalable in performance and infrastructure like you mentioned, and is proven in software in this particular use case. But how can it successfully classify health versus disease patient and identify those biomarkers and those nuances that, Anthoula just came out and shared with us?
Nathan Hayes
Yeah, it’s a really good question and it goes back to what I was mentioning earlier in regards to, the training process versus the inferencing process. The McGill use case that we did with Anthoula, we analyze the data, using a method called K folds, which is basically where you take all of the data you have available and you basically partition it into K different folds where K could equal 5 or 10 or whatever number.
And the idea is that you set aside some of those folds or testing data and the rest of the data is used to train the system. And then after you’ve performed that training, then you set aside a different set of the folds for testing, and then you train again. And so this is a way of training the system, measuring capabilities in the field.
What we realized in this particular use case is that every time every single fold that we did, the training was always a hundred percent. And that was really important because therein lies the evidence of the hypothesis that, Anthoula and the researchers have that there really is a pattern in the data here that ALIX, because of the guarantees that it provides mathematically, based on the unique way that it finds solutions. It’s a proof performed inside the computer of the training solution. And so that is important to let the researchers know that they’re on the right path here, that there’s validation to their thought process. But in addition to that, the one other thing Anthoula mentioned is the ranking of the biomarkers and because of the Modal Interval Arithmetic method that we use with ALIX to solve the training as a by-product or as an outcome of those trainings with the ALIX software or method, we had a ranking of all the different proteins and, we analyzed thousands of proteins and out of all of those proteins, ALIX was able to rank them from the most important to the least important so that we could create a pie chart or a graph that we could provide with the researchers and actually identify by name what were the relative importance of all of these different proteins. And this again is all happening in a non-statistical manner. Basically, it’s a computational proof done inside the computer based on set theory that based on the data and the model that we created, this is the result.
Even though we still have work to do in terms of broaden the database of samples to improve the overall test accuracy of ALIX out in the field and we believe that’s going to improve with time. One of the things that we demonstrated with the K folds testing is that the ranking of the biomarkers did not change hardly at all, between all of the different folds. And so in that sense, you have a high degree of confidence that this list of biomarkers or that signature that Anthoula was talking about is not going to change even as the size of the training database grows over time.
Maria Palombini
It’s just amazing what this technology can do.
Anthoula Lazaris
You can just add to that. So the ranking in science is I can’t stress how important that is, but, ALIX also identified irrelevant protein markers. So you figured, okay. That’s the garbage, it’s not because when we talk about validation, like in a trial and you’re going across multiple different sites, different countries- how do you normalize your data? And that is a major issue in any type of clinical tool you’re going to develop, is normalization. So we haven’t yet finalized this, but we’re exploring with Nate, these irrelevant proteins that do not change between our patient samples. Could we use those to normalize data across sites?
So there’s a plethora of information that we’re still trying to understand in ALIX’s solution.
Maria Palombini
That’s amazing. I think, next time, if ALIX can talk, we should invite him to come to the conversation as well.
All right. I tend to do this to my guests. I’d like them to think quick and have a short, quick answer. So we’ll do this one at a time. So Anthoula, I’ll start with you. When I use the term, or I say the words, “AI for Good Medicine,” what’s the first thing that comes to mind and why?
Anthoula Lazaris
For me, good medicine, just first of all, is meaning improve patient care. So AI for improved patient care to mean means tools or technologies that support patient care.
Maria Palombini
That’s how I envision it as well. Nate, how about you?
Nathan Hayes
For me, I come at it from a little bit different of a perspective and that’s due mainly to my background as the technologist and the mathematician. But to me, the one word is ethics and using the AI in a responsible manner.
Maria Palombini
Absolutely. That’s leading into my next question for Anthoula. You touched on a little bit on this term validation but we often hear about ethics in AI and machine learning for healthcare, and it’s being used in multiple different ways and things, but given your experience now with this particular use case and having to use the application and seeing some of the outcomes and opportunities with it, what would you like to share with the global healthcare community about using these kinds of tools like AI or machine learning that perhaps they may not be aware or even misled when it comes to potentially having real impact in improving patient outcomes?
Anthoula Lazaris
When we look at the ethics component, there’s two things.
There’s data from the patient protecting the patient’s data. And there’s also ethical bias in terms of different patient populations. If we look at data protection in order for us to do the work that we just talked about today with Nate is on our side, on the hospital side. And on the resource side, we had to have ethics protocol to collect the data, but our ethics board and our protocols are very clear.
Any information I provide Nate or I put into any bioinformatics or AI software or technology, they cannot contain any identifiers. These are very well-defined in the ethics community- a date of birth, a day of surgery, names, of course, by far are completely out of this. And all of our data is actually double-coated.
You may ask why don’t you just anonymize? If you want to follow up on these patients to see if you find something interesting. If they’re anonymized, that means you can never go back to follow up on these patients. If they’re double-coated though, and this comes down to another ethics issue is if they’re double-coated and you identify for example, a disease that was maybe a susceptibility to Parkinson’s, et cetera, there are in your ethics protocol and in the consent of this patient there’s procedures put in place that you can actually go back to the patient’s doctor and let the doctor make that decision. That’s just a small example of one of the components that’s embedded in ethics. Our ethics in Canada, I can speak for, and specifically in Quebec, Quebec is actually more restrictive than the rest of Canada. It really protects the patient’s information and I think the patients need to be aware of that, but we can’t overprotect and not be able to go back to the patient to provide their doctors valuable information either. So we have to be aware that we still need to have that openness to go back to the patient when we need to.
With respect to bias, we work in colorectal cancer liver mats, by far it used to always be more male dominating in the older age groups. Unfortunately now with an increase in obesity, we’re seeing a shift in the younger population, but when we do select our patients like you do for a clinical trial, you are biasing your study based on who you know will benefit.
But I think what’s important is like you do in a clinical trial, you need to very, well-define your patient cohorts, the data you’re putting into it. So you already know that it’s going to be biased in what the bias implies. From my perspective, that’s the main two ethical issues.
Maria Palombini
Yes, very important. In blockchain, the quality of what you put in is the quality of what you’re going to get out. It’s almost the same concept and I think it’s really important.
We talk to technologists, they all have a whole array of things that comes to mind when it comes to a challenging aspect or gap that they’re finding in really driving the trust, the adoption, the mainstream acceptance, whatever you want to call it, you know, for the use of the technology in these applications. I guess my question to you is if you had to think of the single most challenging one that’s currently maybe not addressed in current discussions around AI or maybe just keeps getting pushed to the side, creating that little bit of uncertainty on credibility or trust in the tools, what would it be? And in your opinion, what may be one of the best ways to try to resolve it?
Nathan Hayes
Very good question. From a technology perspective, I think the main issue there is about leaving this paradigm of statistical probability behind into the third wave with ALIX and the guaranteed outcomes. But I think more broadly, even in a non-technical manner, I think the most important issue, there is something that Anthoula already touched on a little bit earlier, and that is the interdisciplinary nature that’s required for these programs and I think successful outcomes.
In my own personal view, this is one of the reasons our collaboration with McGill has been so successful is because of the way that our teams have worked together. Bringing our respective areas of domain expertise to the table through dialogue and discussion, being able to overcome the language barriers, so that we can really understand where each other’s coming from.
So we can really understand the medical hypothesis so that we can translate that into a machine learning hypothesis. So that we can take the machine learning results and translate that back into the domain of the medicine and the healthcare. It seems obvious, but the reason I point this out and answer it is because we do actually run into a lot of other scenarios, use cases, people just throw the data over the wall, kind of mentality.
And I think some of that’s just because these domains of technology and medicine are so far apart, it can be a daunting task to overcome that gap. But I think that there’s a lot of that going on. I get worried and concerned about that sometimes in terms of how is that really affecting the work and the quality of the results that are being arrived at using these techniques or methods?
Maria Palombini
Definitely something to think about. You both have shared such tremendous insight today. Any final thoughts that you would like to share with our audience, a call to action or something to get involved or take the extra step, whatever it might be in this pursuit of using these types of technologies to really start making an important impact in the area of precision oncology and research and that kind of thing?
Anthoula Lazaris
First and foremost communication, communication, communication. Like Nate just mentioned being able to understand each other’s language when you don’t know something, say you don’t know it and bring in others to help support you. I think that’s one key thing. And like Nate said, I think that’s why we’ve succeeded in what we’re doing so far.
And a quote. I can’t remember who said it, but basically it’s not enough to just do our best, but we need to know what to work on. With this specific example, we had one question, one hypothesis, and we got a solution. I find in science sometimes people are over-ambitious. They say, wow, ALIX is amazing.
They’ll try to feed it a whole bunch of data, but you need to stay focused and you need to have a simple question. Like Maria, you said at the beginning, we want pragmatic to be pragmatic. We want to be able to allow our patients to receive these solutions. In order to be pragmatic, we need to ask simple questions.
Maria Palombini
Very good point. And Nate, how about you?
Nathan Hayes
I would really like to just follow on that and add my second to it. It’s just so important to emphasize. I really do believe it is the most important thing to end on here that as exciting as all of these technologies are particularly ALIX and the new capabilities that it brings to the table, the machine learning and the AI, it is still just a tool. Everything in terms of the quality of the outcomes, the ethical nature really depends on the humans that are using the technology and how they work together.
Maria Palombini
That’s fascinating and very good parting points for our audience.
Many of the concepts that we’ve talked about today with Anthoula and Nate are currently being addressed in various activities here, IEEE SA Healthcare and Life Science practice. We cover a lot of areas of blockchain, AI, quantum, forward-thinking in mobile healthcare, telemedicine, whatever it takes to improve the patient outcomes across the healthcare value chain.
So we will include the links to Modal Technology Corp and the Research Institute at McGill University on the blog posts that’ll be accompanying this podcast. You can learn more about these respective organizations and the great work they’re doing.
Please check out the Healthcare and Life Science practice website at ieeesa.io/hls. We’ll have all the information about the different incubator programs we’re doing. They’re open for everyone to participate and to help us contribute towards global solutions to try to drive responsible and validated adoption of these technologies. I ask all of you to please, if you liked this podcast, please share it on your networks and actually use hashtag #IEEEHLS, or you could tag me Maria Palombini or the IEEE Standards Association. So we can give everyone access to this great information and this awesome case study. We want to get it out there and make everybody aware of what’s going on. I want to say a special thank you to Nate and Anthoula for joining us today. Nate and Anthoula, thank you. This was so great.
Nathan Hayes
Thank you.
Anthoula Lazaris
You also for having us.
Maria Palombini
Pleasure. And to all of you in the audience. Thank you for joining us. I want to continue to wish you to stay safe and well, and please keep tuning in as we bring the bright minds, such as the ones we’ve had today to keep sharing these great insights with me and with all of you. Until then take care.